Rectified Gaussian distribution
In probability theory, the rectified Gaussian distribution is a modification of the Gaussian distribution when its negative elements are reset to 0 (analogous to an electronic rectifier). It is essentially a mixture of a discrete distribution (constant 0) and a continuous distribution (a truncated Gaussian distribution with interval ) as a result of censoring.
Density function
The probability density function of a rectified Gaussian distribution, for which random variables X having this distribution, derived from the normal distribution are displayed as , is given by
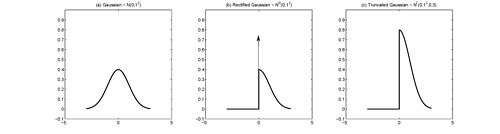
Here, is the cumulative distribution function (cdf) of the standard normal distribution:
is the Dirac delta function
and, is the unit step function:
Mean and variance
Since the unrectified normal distribution has mean and since in transforming it to the rectified distribution some probability mass has been shifted to a higher value (from negative values to 0), the mean of the rectified distribution is greater than
Since the rectified distribution is formed by moving some of the probability mass toward the rest of the probability mass, the rectification is a mean-preserving contraction combined with a mean-changing rigid shift of the distribution, and thus the variance is decreased; therefore the variance of the rectified distribution is less than
Generating values
To generate values computationally, one can use
and then
Application
A rectified Gaussian distribution is semi-conjugate to the Gaussian likelihood, and it has been recently applied to factor analysis, or particularly, (non-negative) rectified factor analysis. Harva [1] proposed a variational learning algorithm for the rectified factor model, where the factors follow a mixture of rectified Gaussian; and later Meng [2] proposed an infinite rectified factor model coupled with its Gibbs sampling solution, where the factors follow a Dirichlet process mixture of rectified Gaussian distribution, and applied it in computational biology for reconstruction of gene regulatory networks.
References
- ↑ Harva, M.; Kaban, A. (2007). "Variational learning for rectified factor analysis☆". Signal Processing. 87 (3): 509. doi:10.1016/j.sigpro.2006.06.006.
- ↑ Meng, Jia; Zhang, Jianqiu (Michelle); Chen, Yidong; Huang, Yufei (2011). "Bayesian non-negative factor analysis for reconstructing transcription factor mediated regulatory networks". Proteome Science. 9 (Suppl 1): S9. doi:10.1186/1477-5956-9-S1-S9. ISSN 1477-5956.